Today let’s look at something I think you shouldn’t be doing with AF extraction in spectral flow cytometry: automated AF extraction with the gate set on everything. This isn’t a strawman argument—I know people who have been told to do this when they received training on a spectral instrument.
I’m not sure what the thinking is behind this approach, but I’d guess it’s something along the lines of “I want to extract the AF from my cells. These are my cells. By including all of the live cells, dead cells, debris and doublets, I’ll remove all of that AF.” That’s not how it works.
Now, I don’t have access to the exact math behind the spectral unmixing for Cytek, Sony or BD’s machines, but they all use a variation of least squares to unmix and appear to use normalized spectral signatures as input.
An un-normalized spectral signature for a weakly expressed GFP
The normalized spectral signature for that GFP. The data have been adjusted to a maximum of 1 in the peak channel, with the values in the other channels expressed as a fraction of the maximum.
What this implies is that when we unmix a single AF channel, we are supplying a single normalized AF signature to the unmixing model in the software. This is not going to be the sum of the autofluorescences in the data, but rather the median. This means the signature is going to be weighted by the distribution of cells/datapoints in the gate; the median will be closer to the most frequent cell type (or debris if you’ve got more debris than cells). Think of it this way: if we have 10 cells with a yellow AF signature and 90 with a blue AF signature, the median AF signature is a composite of the two with a yellow peak at ~11% (10/90) of the blue peak. If we have 50 yellow and 50 blue, we get peaks of equal height. Those are very different spectral signatures.
Importantly, the median of a mixed cell (or cell and debris) population is not going to be representative of any individual type of cell within that population. There’s a famous example of this problem from the US Air Force in a book by Todd Rose (article here) on the work of Gilbert Daniels. Out of over 4000 pilots, not one conformed to the average dimensions of the group. As a result, an average-sized cockpit wasn't a good fit.
Okay, back to cytometry stuff. Just like any other fluorophore, we need to be sure that the information we are feeding into the unmixing model is representative of the data we are trying to analyze. This is where we need to think about what we want to accomplish. Are we trying to exclude or extract the autofluorescence from debris or dead cells? Probably not. If we have an FSC/SSC gate, we already have a better exclusion method for debris. Similarly, if we have a viability dye (you’d better have one!), we’re already excluding dead cells, so including dead cells in the AF calculation can only throw it off. The same goes for doublets or large cells that get excluded by gating. Anything that’s being excluded effectively by your gating strategy isn’t going to be the right AF signature in the data you’re actually analyzing.
Let’s have a look at an example. For this, I’ll be using a messy dataset I generated for the Babraham Spectral Symposium. You can access the data at Mendeley Data (I find Flow Repository really hard to use these days). This is not amazing data, and that’s the point. It’s a mouse surface staining experiment with spleen, lung and brain, all of which have distinct cell populations capable of causing AF problems. The staining set is designed to highlight issues with unmixing and extracting AF, as we saw in this post on control clean-up.
Let’s focus on the brain AF. In the brain, we have a substantial population of microglia, a specialized macrophage type that exhibits broad-spectrum AF. If we don’t extract AF, we get these sorts of problems in the data:
CD11b+ microglia showing unmixing errors. These particular errors are fixable with AF extraction.
In these mice, the iCas9-GFP is expressed only in T cells through the action of a CD4Cre cleaving the flox-stop flanking sequences. Microglia also don’t express CD4 of much/any B220 (at least, under homeostatic conditions). Nothing should be skewed negatively.
If we gate on everything, like so:
The unmixing changes to look like this:
Yes, there are improvements, particularly for GFP and B220-SBUV445, and probably a bit for CD4-BV510. We’ve extracted an AF signature with peaks in those areas, which is a pretty generic signature. There’s still a skew versus IgD-BUV496, and the GFP, CD4-BV510 are still off.
Why isn’t this working well? If we set our AF extraction with a gate specifically on regions where the microglia aren’t, such as the debris/dead cell area, the skews are worse. This makes sense, right? If we aren’t feeding a microglia AF spectrum into the unmixing, the unmixing can’t correct it properly.
Similarly, gating on very large high SSC events also produces inaccurate AF extraction for the microglia, which aren’t up there.
If we instead focus the AF gate on the FSC/SSC region where we expect to find microglia, we can get better unmixing for the microglia, as follows:
Not all the errors in the data are fixed with this gating of the AF. This is a simple method, and there are more problems than just AF in these data.
Here’s the spectra of those regions:
The general shape of the curves is similar, which is why the AF extraction is somewhat effective no matter where we set the gate. The devil is in the details, though. Moreover, we can see that as we focus in on the microglia region, we start getting two traces. That upper trace is the microglia, while the lower one is other, less autofluorescent cells in the same region such as monocytes, lymphocytes, astrocytes and oligodendrocytes. As we’ve seen in posts on cleaning up cell controls here and here, these sorts of messy signatures don’t generate great unmixing because there is uncertainty. To get a clean signature of microglia or any other cell population that can’t be gated to isolation by scatter (probably most cell types except maybe monocytes in human PBMC), we need different approaches. The AF explorer tools (Cytek, ID7000) are one approach and we’ll look at this in the future.
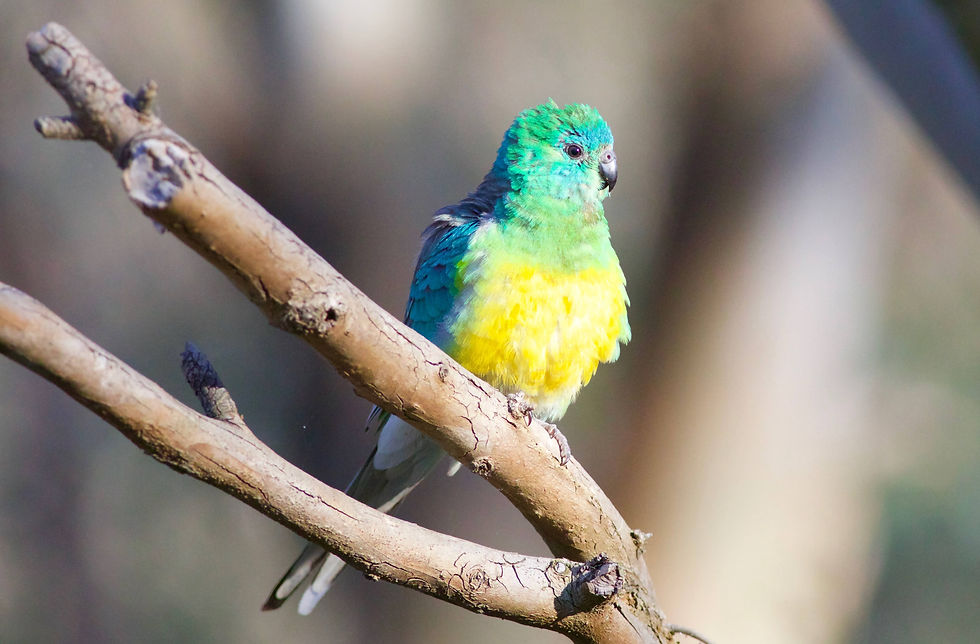
Comments