Backgating is a simple, useful technique in flow cytometry that can help you figure out where your cells are, and thus optimize your gating strategy.
Let’s say we’re looking for a rare, activated T cell, such as the example here with peptide-responding antigen-specific T cells.
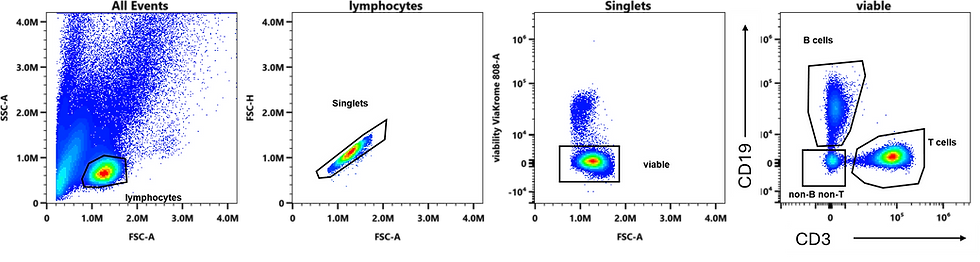
Human PBMC in an Antigen-Induced Marker (AIM) assay. For the gating strategy here, I'm using the unstimulated control, gating tightly around the population to get what I think will be clean data.
I can identify the cells in a standard gating process, starting with scatter, then singlets, dead cell exclusion, CD3+ T cells, then my antigen-reactivity markers CD154 and CD69. Here's what that looks like:
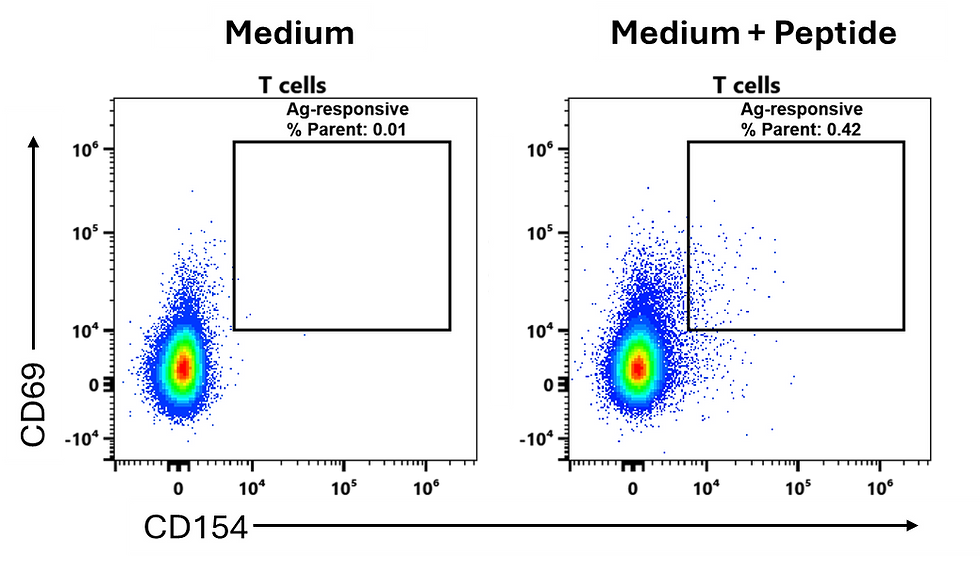
CD69 and CD154 upregulate after the cells are re-stimulated with peptide. Not bad.
But, how do I know I haven’t lost any cells along the way? I think my CD154+ T cells will be in the same position as my other T lymphocytes, but there are good reasons they might not be. For instance, if the CD154+ T cells are activated, the scatter may change. I wouldn’t notice this in the plot of FSC/SSC because I’ve only got about 100 CD154+ T cells out of a million or so total PBMCs. Similarly, if there are any unmixing issues due to the fluorophore I’ve used for the CD154 causing it to spill into the viability dye channel, I wouldn’t see this because there are just too few events. (The CD154 antibody I'm using here has an emission wavelength similar to the ViaKrome 808 viability dye.) I might even expect a change in autofluorescence in the CD154+ cells due to activation-induced metabolic changes.
So, here’s how we figure that out. We take our final gated population and plot it on the axes we’ve used to gate it. Where does the final target population fall in relation to the gate I’ve used to select it? If it’s well within the gate boundaries, no problem. If it’s touching the edge and getting cut off, well we didn’t set that gate wide enough.
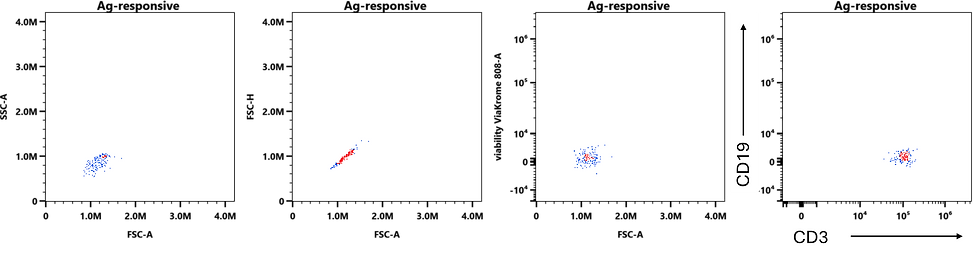
Antigen-responding CD154+CD69+ T cells plotted on the dimensions we've used to identify them. If you use FlowJo, you can drop the gates on these plots for a better visualization. Note the sharp edges to the cell population on the lefthand plot.
In this case, the very first plot reveals a problem. The FSC/SSC gate is cutting into the activated T cell population. This makes sense--we know that T cells increase their SSC when activated. It's also not clear whether all the cells are making it through the viability gate, so let's check that, too.

Extending the viability gate doesn't change the final percentage of Ag-responsive T cells. We aren't disproportionately removing our target cells with this gate.
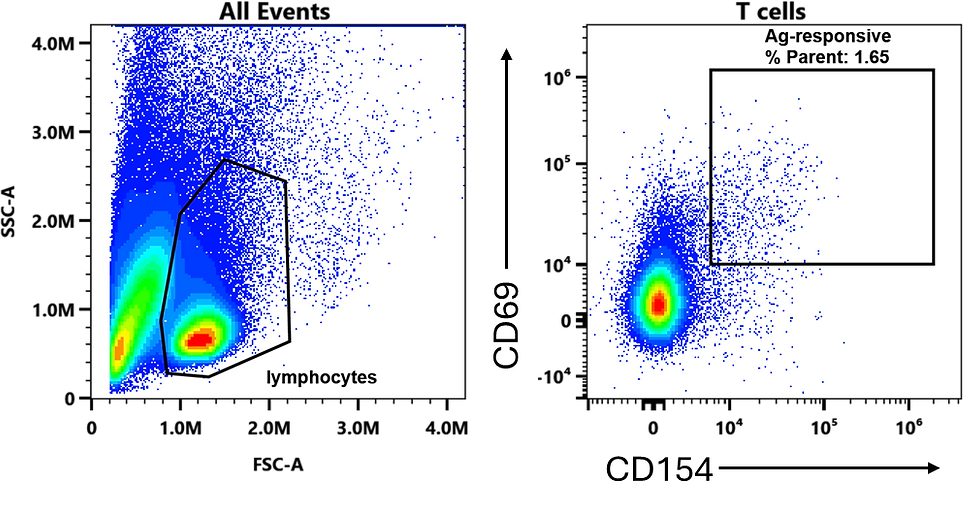
Expanding the FSC/SSC gate radically alters the frequency of Ag-responsive T cells. The tight gate was preferentially removing our target cells.
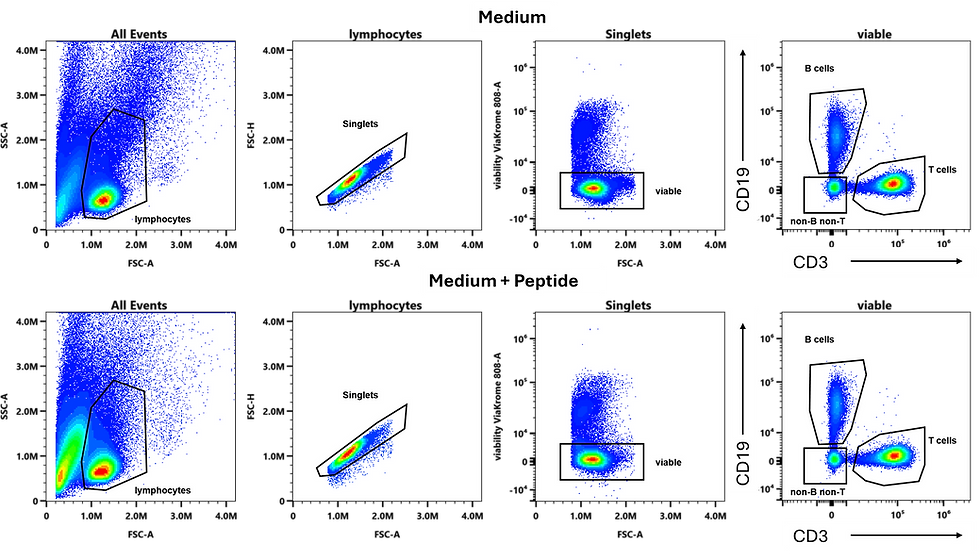
Final gating strategy after performing backgating checks. Top row: unstimulated. Bottom row: peptide-stimulated. Note how we can't really pick out the rare antigen-responsive T cells in the first FSC/SSC gate.
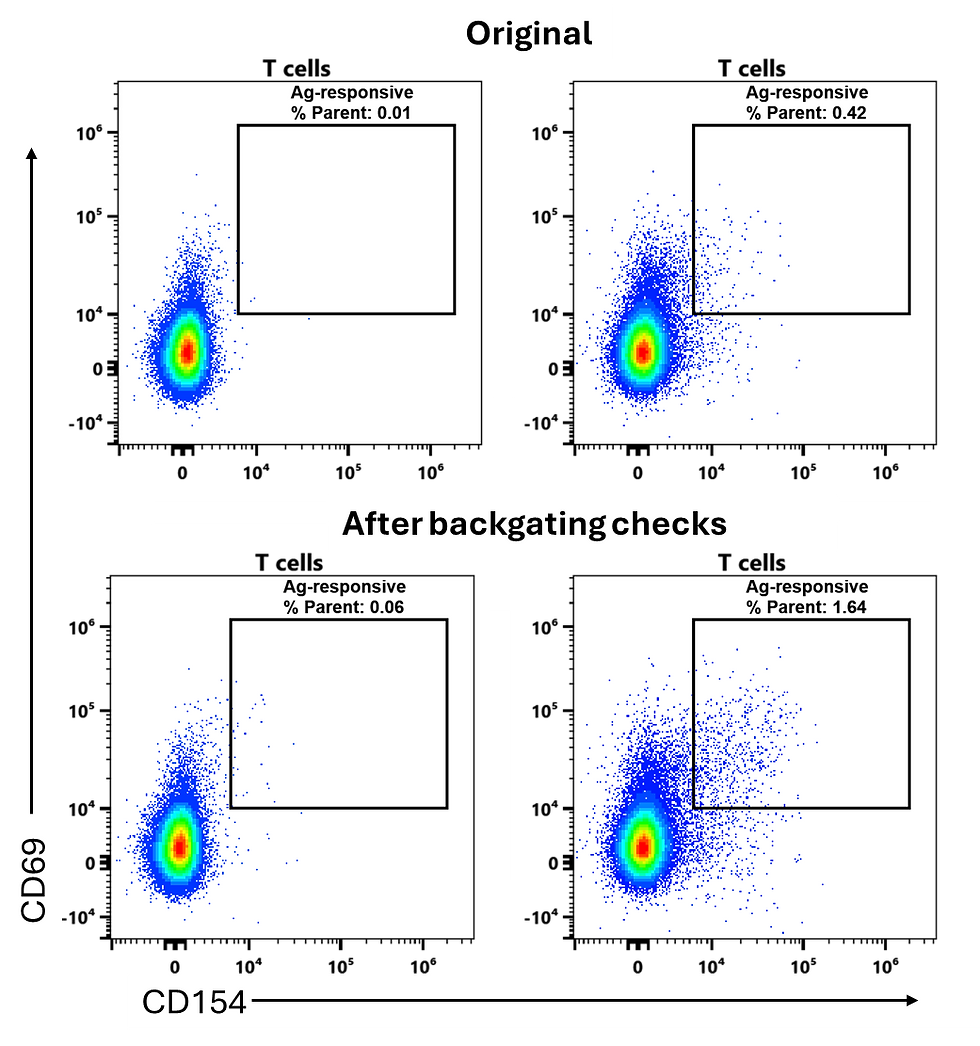
Comparison of the AIM results before and after performing backgating to optimize the gating strategy.
Alternatively, if at any point the plot starts to reveal multiple populations, we need to assess what those populations are and whether we should be including them in the gate, or if we should be adjusting the gate to exclude them. This can be a problem with monocytes or other myeloid cells creeping into your gating strategy due to autofluorescence and non-specific binding. In those cases, the issue can often be fixed by adjusting the scatter gate. If that doesn’t work, you may need to modify your panel to either improve the separation of key gating markers or to include an exclusion gate (e.g., CD14 for monocytes, F4/80 for macrophages). This is sort of similar to the process illustrated here for cleaning up controls to remove intrusive autofluorescence.
The need for backgating illustrates a general problem with using gating on 2D plots of multidimensional data. We can't see the rest of the data, so we don't know if what we're doing is really the best choice. Gating in 2D works fine for 2D data from the FACScan (and it's pretty good for 3D and 4D data as well). As we get into larger and larger panels, finding an optimal gating strategy becomes more difficult. One way around this is to use clustering, which comes with its own set of issues. We should really be able to gate in multiple dimensions simultaneously, and projects such as Ryan Brinkman's SOULCAP and Jonathan Irish's Velociraptor offer hints of how this sort of approach might work. Anyway, this is probably fodder for another discussion.
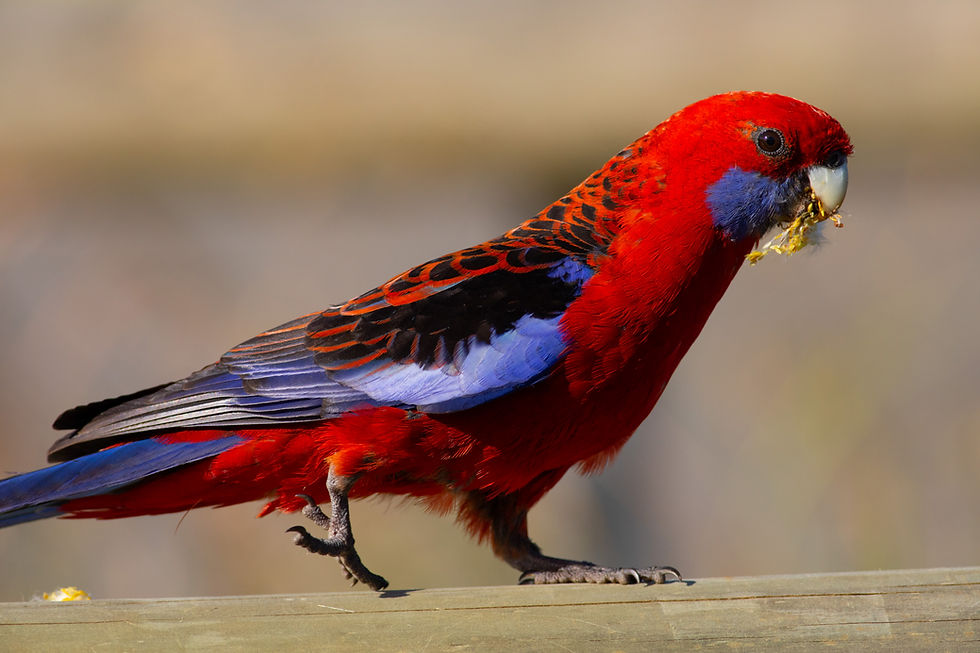
Crimson Rosella, Australia
Comments