In this series on autofluorescence (AF), I’ll post some musings on how AF affects flow cytometry data and approaches for dealing with it. I aim to look at:
Classification of AF problems
What are we trying to achieve by correcting for AF
AF and panel design
Methods for dealing with AF in conventional flow
What not to do with AF extraction in spectral flow
Current methods for AF extraction in spectral flow
Reducing background
Eliminating noise
Correcting multiple cell populations
Correcting multiple tissue sources
AF and implications for bead vs. cell controls
Thoughts on how we might better deal with AF
At the moment, we’re in the Wild West with AF extraction. We’ve figured out that it’s important and we suddenly have some tools to deal with it. These tools differ depending on the cytometer and software, and in many cases, the user. That's not a great situation for standardization or reproducibility.
To be clear, I do not claim to have the answers here, just some thoughts. I’d love it if it so happens that five years from now, most of what I say here is out of date or proven incorrect by advances in our knowledge. As usual, I’ll try to back up what I’m saying with examples from real data, but as we’ll see, AF and the best way for dealing with it depends on the data set, so my examples are not going to be universal truths.
Let’s start today by recognizing that AF is not a monolith. There are different types of challenges we deal with that fall under the headline “Autofluorescence”. Let’s clarify the different types of issues we face that stem from AF in flow cytometry. To my mind, these are the different types of problems, and these require different solutions. This isn't to say that the underlying causes are different, but how we deal with the manifestations may be different. This list probably isn't comprehensive, but it's a start.
Types of problems caused by AF in flow cytometry data:
Background. A general increase in signal across multiple channels in most or all cells. This can be caused by the buffers or fixatives being used.
Note how the general profile is similar, but the trace is higher across most of the spectrum after fixation and permeabilization, in this case using the eBioscience Foxp3 kit.
Noise. Spikes of cells, traditionally going up diagonally through the middle of biplots.
There is no staining here, just AF.
Cell-type specific inaccuracy. An incorrectly unmixed/compensated cell population or populations. Here we are talking about something that tracks with the cell type across multiple fluorophores, not unmixing issues due to poor identification of the spectra.
A sample of lung cells stained only with Siglec F BUV615 (y-axis). There are multiple Siglec F+ cell populations in the lung with distinct AF profiles, at least one of which has not been corrected here using the automated AF extraction in SpectroFlo.
Tissue/source-specific inaccuracy. A difference in the background and/or cell types between conditions (e.g., tissues, fixatives). This is basically the same as above, but if we're trying to compare across conditions with and without the problem population, the solution may be different.
A comparison of murine spleen and brain stained with the same set of antibodies (unoptimized) and unmixed rather crudely with automated AF extraction in SpectroFlo. The brain contains a population of CD11b+ microglia with a distinct AF signature, leading to microglia being artefactually positive or negative across multiple channels if this is not corrected for.
Note that there are probably other issues here, such as the lean between BUV496 and BUV805 that indicates inadequate blocking with Brilliant Stain buffer, at least for the brain sample. This is a problematic data set I'll use for examples in this series.
Control interference. Interference with identification of spectra for compensation or unmixing. This is the problem dealt with in this post and this one.
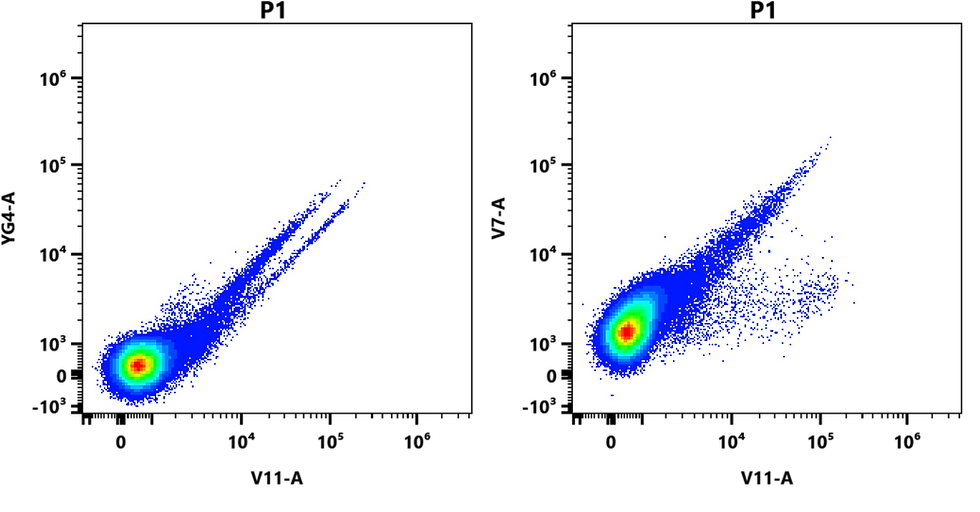
Example of a single-stained control with lots of AF noise in the same part of the spectrum in the raw space. On the right, we can see the real signal appearing in V11 with the noise going up as a correlation between V11 and V7.
Loss of signal. Interference with the measurement of a specific fluorophore (e.g., GFP).
In this example, we're looking at the raw data, focusing on the B1 detector where GFP has its peak emission. On the lymphocyte population, we can see the signal of our (weak) GFP reporter in the transgenic animal (right) versus the WT (left). In the brain or the granulocytes of the spleen, however, there is already more signal in this channel in the WT animal (bottom row) than in the real GFP sample.
Unmixing distortion and inaccuracy. These problems arise due to our attempts to correct for AF using suboptimal techniques and due to failure to account for AF/AF extraction in our panel design process.
In these examples, the AF extraction is creating a substantial distortion in our data, with a loss of resolution and, in the bottom example, leading to the inability to separate positives from negatives.
Over the next few weeks, we can dive into these issues and try to address how best to resolve them.
Food for thought: All of the approaches we currently have to extract, unmix or compensate AF operate under the assumption that the signal varies in intensity but not quality, like the fluorophores we use for marker detection. This is not correct. In a massive over-simplification, AF arises from cellular metabolism, differentiation and activation status; as such, there are gradients of many AF-producing molecules along these axes, producing distinct but similar combinations in most cell types and states. In order to actually get correct unmixing of spectral flow cytometry data, we need a much more granular assessment and correction of autofluorescence signatures.
Looking forward to this series! I work on granulocytes in the mouse lung and feel like I will learn a lot from this dive into AF